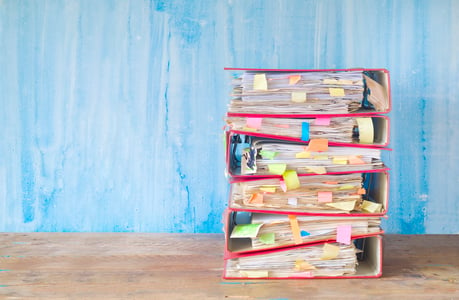
Data. Data. Data.
We hear a lot about using data these days. We know it is important and useful, but honestly, it can be overwhelming. So, how do you get started?
Let’s begin with some definitions to help you understand what CLASS data is, how to collect it, and other topics such as validity and reliability. These topics are all important when designing or improving a CLASS data collection system.
CLASS Data
The first step you should take when approaching CLASS data is to understand what data you are collecting in the classroom and how you should go about collecting it.
What is CLASS data?
Data is another word for information. When we talk about CLASS data we generally mean the dimension and domain scores that come from coding a classroom’s teacher-child interactions.
We can also gather important data from the observation, such as the time of day, the number of teachers and children in the classroom, the type of activities, and the content areas addressed.
Information about what was happening during the interactions can help us interpret the scores and provide meaningful professional development to teachers.
Why should I collect CLASS data?
Collecting and using data helps us make more accurate decisions about what is happening in classrooms, both where to celebrate teachers’ strengths and how to support teachers’ growth.
Data gives us:
- a starting point or baseline,
- a roadmap for growth,
- a way to compare across classrooms and programs,
- and a measure of success (i.e., did we accomplish what we set out to do).
Validity
Now that we know what we are trying to collect and what we can do with it, we can turn our attention to how to make sure that the data we collect is valid.
What is validity?
Validity looks at if a tool measures what it is supposed to measure. Imagine you are aiming at a target. In the picture below, the target on the far left shows that you hit a spot multiple times, but not the bullseye. The target on the far right shows that you hit the bullseye; you got what you were aiming for.
Validity can be confusing because there are many different types, and each captures a different aspect of the data collected by the tool. Let’s take one type as an example: predictive validity looks at if the tool predicts the outcomes you hope it will predict. In the case of the CLASS, this means that we want the interactions captured by the CLASS to predict children’s social and academic outcomes.
Why is validity important when using the CLASS tool?
We care about validity because we want to use a tool that captures aspects of the classroom related to child outcomes. We also want the tool to measure interactions accurately. More than 200 empirical studies confirm the construct, convergent, divergent, and predictive validity of data collected with the CLASS system. Specific information of the types of validity for each age group can be found in the technical appendices within the CLASS manuals.
Reliability
In order to get valid data, we have to think about reliability. You may have heard this term in relation to CLASS observer certification. Let’s dig into what it means to collect data reliably.
What is reliability?
Reliability helps us ensure that the data we capture is free from error. Another way to think about it is consistency; measurement using the CLASS tool should be consistent across observers, classrooms, time of day, etc. There are several types of reliability, but we will focus here on the reliability of the observers.
If we go back to the target picture, the dots on the target that are close together demonstrate reliability (the far left and far right targets). The middle targets are not reliable because the dots are all over the place.
Why is reliability when using the CLASS tool important?
We want the way that we measure interactions to be the same regardless of the observer. If observers are using the tool differently, then it is hard to know if the difference in scores is because of the classroom interactions or because of the observer.
Variance
Before we talk about how to ensure reliability, we need to understand variance.
What is variance in CLASS scores?
Variance is a statistical term, but simply put, it means difference. In CLASS data collection, variance is how much the range of collected CLASS scores differs from the average CLASS score for that set of teachers observed. More variance means that individual scores are farther from the mean and less variance means that individual scores are closer to the mean.
Here’s an example. We’ll look specifically at Emotional Support (ES). In the chart below, we see the distribution for all of the ES scores for a set of programs. The mean score, or average, is represented by the peak of the black line. The variance then is how much all of the other scores in the chart differ from that mean.
Because most of the scores are close the mean score, the variance is relatively small. When we look at Instructional Support (IS), we see that the variance is a little bigger; there are more scores that are farther away from the mean.
Why is it important to understand variance?
Variance is to be expected. Each classroom, teacher, and child are different. When measuring teacher-child interactions for both professional development and high-stakes decisions, we want as much of the variance as possible to be attributed to the teacher-child interactions, rather than observer bias, sending only one observer to assess a whole program, or outside factors such as work environment.
This allows stakeholders, administrators, coaches, and teachers to make more accurate decisions about next steps for policies, programs, and professional development.
Putting It All Together
Now we are back to collecting reliable data. How do all of these ideas connect and how do I get started?
How does variance relate to collecting CLASS data?
When data is collected reliably (meaning in the same way each time across observers), you are able to control for most of the variance outside of the actual interactions observed. Additionally, decisions such as number of cycles per classroom and number of classrooms observed can affect how much variance you capture and how you much you can infer from the data.
For example, the more cycles you are able to obtain and average at the program level, the more stable the estimate of typical classroom interactions will be for that program.
How do I ensure reliability?
There are several ways to ensure reliability when collecting data:
- Use only certified observers to collect CLASS observation data.
- Have clear a protocol for observers and provide training on the protocol.
- Have observers complete calibration videos to compare their coding with master codes.
- Include double coding observations (where two coders observe and score simultaneously).
- Complete fidelity checks to ensure adherence to protocols.
There you have it, a bunch of complicated concepts that boil down to the idea that we want to measure teacher-child interactions because they matter for kids, and we want to do that in a way that helps get precise and accurate data so we can make the best decisions based on that information.
And here’s the best news!
Teachstone is currently working on several ways to make designing and implementing CLASS data collection a little bit easier and a lot more effective. We are creating a series of webinars, guidance documents, and sample protocols to provide support to help you think through specific data decisions for your data collection system.